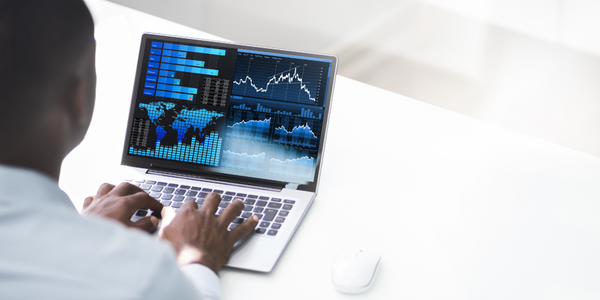
技术
- 分析与建模 - 机器学习
- 分析与建模 - 预测分析
- 功能应用 - 远程监控系统
- 平台即服务 (PaaS) - 数据管理平台
- 传感器 - 温度传感器
适用行业
- 药品
适用功能
- 维护
用例
- 预测性维护
客户
未公开
关于客户
该公司是一家总部位于美国的领先生物技术制造商,使用尖端的专有技术开发和制造营养成分。公司自成立以来发展迅速,产生了令人印象深刻的
挑战
为了提高生产能力并避免停机,一家全球生物技术制造公司实施了 Seebo Predictive Analytics。
该公司的季度运营评估显示,生产期间的停机时间增加了 3.6%。这种停机时间源于生产线中一种产品的无法解释的粘度。
由此产生的反应器和生产线离心机之间的管道堵塞导致批量生产过程中更频繁的设备清洁程序和停机、高水平的浪费、产能下降和上市时间延长。
调查小组无法确定堵塞的原因,因为所有相关的生产参数都在批准的工作范围内。
解决方案
该公司决定投资工业 4.0 和预测分析,并寻找具有以下功能的解决方案:
- 将他们的制造专业知识与数据分析和机器学习相结合
- 为运营团队提供简单而准确的见解
- 预测未来的停机问题
Seebo 分析了生产线的历史数据和在线数据,并确定了导致堵塞的变量之间的相关性——混合持续时间、蒸馏时间和反应温度的具体变化。
基于这些发现,Seebo 解决方案可以在再次发生堵塞之前向运营团队提供预测警报。
借助 Seebo 解决方案,工厂恢复了预期的生产能力,生产团队能够确定正确的预测性维护计划。
收集的数据
Duration, Distillation time, Reaction temperature
数量效益
Case Study missing?
Start adding your own!
Register with your work email and create a new case study profile for your business.
相关案例.
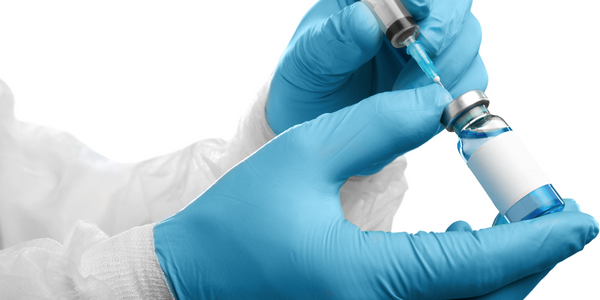
Case Study
Case Study: Pfizer
Pfizer’s high-performance computing software and systems for worldwide research and development support large-scale data analysis, research projects, clinical analytics, and modeling. Pfizer’s computing services are used across the spectrum of research and development efforts, from the deep biological understanding of disease to the design of safe, efficacious therapeutic agents.
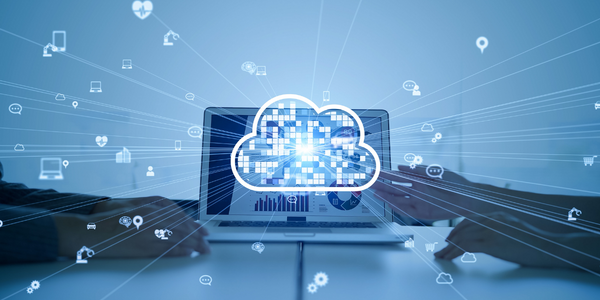
Case Study
Fusion Middleware Integration on Cloud for Pharma Major
Customer wanted a real-time, seamless, cloud based integration between the existing on premise and cloud based application using SOA technology on Oracle Fusion Middleware Platform, a Contingent Worker Solution to collect, track, manage and report information for on-boarding, maintenance and off-boarding of contingent workers using a streamlined and Integrated business process, and streamlining of integration to the back-end systems and multiple SaaS applications.
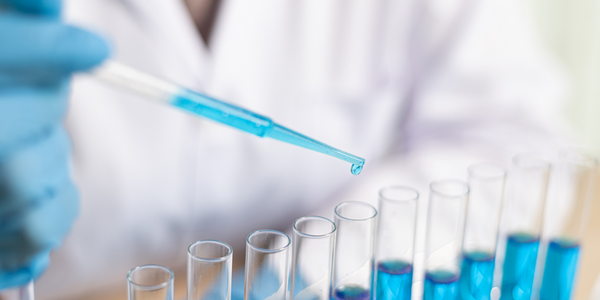
Case Study
Process Control System Support
In many automated production facilities, changes are made to SIMATIC PCS 7 projects on a daily basis, with individual processes often optimised by multiple workers due to shift changes. Documentation is key here, as this keeps workers informed about why a change was made. Furthermore, SIMATIC PCS 7 installations are generally used in locations where documentation is required for audits and certification. The ability to track changes between two software projects is not only an invaluable aid during shift changes, but also when searching for errors or optimising a PCS 7 installation. Every change made to the system is labour-intensive and time-consuming. Moreover, there is also the risk that errors may occur. If a change is saved in the project, then the old version is lost unless a backup copy was created in advance. If no backup was created, it will no longer be possible to return to the previous state if and when programming errors occur. Each backup denotes a version used by the SIMATIC PCS 7 system to operate an installation. To correctly interpret a version, information is required on WHO changed WHAT, WHERE, WHEN and WHY: - Who created the version/who is responsible for the version? - Who released the version? - What was changed in the version i.e. in which block or module of the SIMATIC PCS 7 installation were the changes made? - When was the version created? Is this the latest version or is there a more recent version? - Why were the changes made to the version? If they are part of a regular maintenance cycle, then is the aim to fix an error or to improve production processes? - Is this particular version also the version currently being used in production? The fact that SIMATIC PCS 7 projects use extremely large quantities of data complicates the situation even further, and it can take a long time to load and save information as a result. Without a sustainable strategy for operating a SIMATIC PCS 7 installation, searching for the right software version can become extremely time-consuming and the installation may run inefficiently as a result.
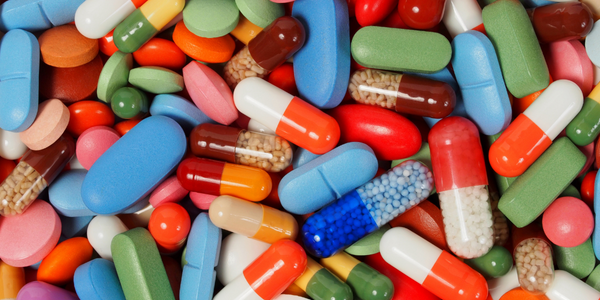
Case Study
Drug Maker Takes the Right Prescription
China Pharm decided to build a cloud-based platform to support the requirements of IT planning for the next five to ten years which includes a dynamic and scalable mail resource pool platform. The platform needed to have the following functions: all nodes support redundancy, ensuring service continuity and good user experience, simple and easy-to-use user interfaces for end users and administrators and good compatibility and supports smooth capacity expansion.
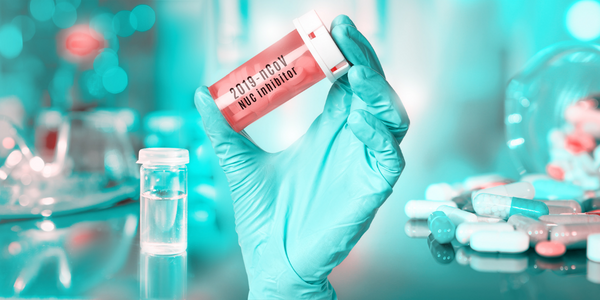
Case Study
ELI LILLY ADOPTS MICROMEDIA’S ALERT NOTIFICATION SYSTEM
Pharmaceutical production is subject to a strict set of enforced rules that must be adhered to and compliance to these standards is critically necessary. Due to the efforts of WIN 911’s strategic partner Micromedia, Lilly was able to adopt an alarm notification infrastructure that integrated smoothly with their existing workflows and emergency hardware and protocols. These raw energy sources enable the industrial process to function: electricity, WIN-911 Software | 4020 South Industrial Drive, Suite 120 | Austin, TX 78744 USA industrial steam, iced water, air mixtures of varying quality. Refrigeration towers, boilers and wastewater are monitored by ALERT. Eli Lilly identified 15000 potential variables, but limitations compelled them to chisel the variable list down to 300. This allowed all major alarms to be covered including pressure, discharge, quantity of waste water discharged,temperature, carbon dioxide content, oxygen & sulphur content, and the water’s pH.