公司规模
1,000+
地区
- America
国家
- United States
产品
- DataRobot’s Enterprise AI platform
- DataRobot’s AI Services
技术栈
- Machine Learning
- Predictive Models
实施规模
- Enterprise-wide Deployment
影响指标
- Digital Expertise
- Productivity Improvements
技术
- 分析与建模 - 预测分析
- 应用基础设施与中间件 - 数据交换与集成
适用行业
- 金融与保险
适用功能
- 商业运营
用例
- 欺诈识别
- 预测性维护
服务
- 数据科学服务
关于客户
客户是一家总部位于美国的领先金融科技公司。他们帮助各行各业的客户获得购买商品和服务所需的资金和付款计划。他们还帮助商家和服务供应商通过信贷计划增加销售额。该公司使用机器学习模型进行决策,这在受到严格监管的金融行业中至关重要。他们在 DataRobot 平台上构建了多个模型并部署到生产中,包括内部信用评分模型、欺诈评分模型和经销商评分模型。
挑战
这家总部位于美国的金融科技公司在将其业务流程与监管合规要求相一致方面面临挑战。他们使用机器学习模型进行决策,由于该行业受到严格监管,这增加了风险。该公司已经在使用 DataRobot 的企业 AI 平台来改进其模型构建,但他们需要加快其业务流程与模型风险管理法规的一致性。他们在 DataRobot 平台上构建了几个模型并部署到生产中,包括内部信用评分模型、欺诈评分模型和经销商评分模型。然而,在与银行合作后,他们需要进行独立的模型验证,这是他们合作的关键组成部分。
解决方案
该金融科技公司与 DataRobot 的 AI 服务部门合作,独立验证其模型。DataRobot 的 AI 服务团队独立于公司其他部门运营,独立完成了验证工作的五个主要阶段:项目规划、文档和方法审查、模型性能、测试和分析以及最终模型验证报告和工作底稿的准备。该团队提供了金融科技公司所需的独立验证,并附有模型验证报告,该报告详细描述了模型的工作原理,以及在验证过程中为有效挑战该模型的开发所做的工作。该公司计划每年与 DataRobot 的 AI 服务部门合作,以满足其模型验证需求。
运营影响
数量效益
Case Study missing?
Start adding your own!
Register with your work email and create a new case study profile for your business.
相关案例.
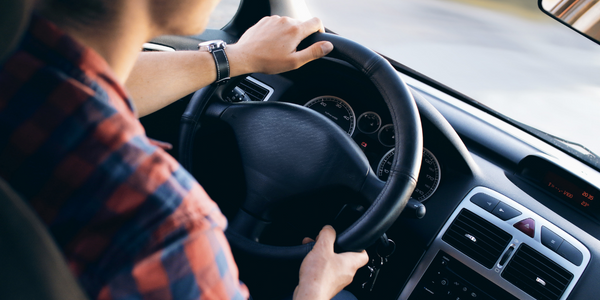
Case Study
Real-time In-vehicle Monitoring
The telematic solution provides this vital premium-adjusting information. The solution also helps detect and deter vehicle or trailer theft – as soon as a theft occurs, monitoring personnel can alert the appropriate authorities, providing an exact location.“With more and more insurance companies and major fleet operators interested in monitoring driver behaviour on the grounds of road safety, efficient logistics and costs, the market for this type of device and associated e-business services is growing rapidly within Italy and the rest of Europe,” says Franco.“The insurance companies are especially interested in the pay-per-use and pay-as-you-drive applications while other organisations employ the technology for road user charging.”“One million vehicles in Italy currently carry such devices and forecasts indicate that the European market will increase tenfold by 2014.However, for our technology to work effectively, we needed a highly reliable wireless data network to carry the information between the vehicles and monitoring stations.”
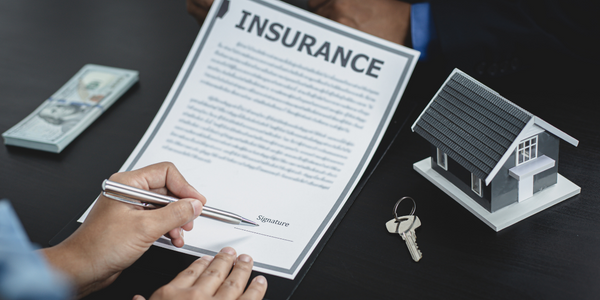
Case Study
Safety First with Folksam
The competitiveness of the car insurance market is driving UBI growth as a means for insurance companies to differentiate their customer propositions as well as improving operational efficiency. An insurance model - usage-based insurance ("UBI") - offers possibilities for insurers to do more efficient market segmentation and accurate risk assessment and pricing. Insurers require an IoT solution for the purpose of data collection and performance analysis
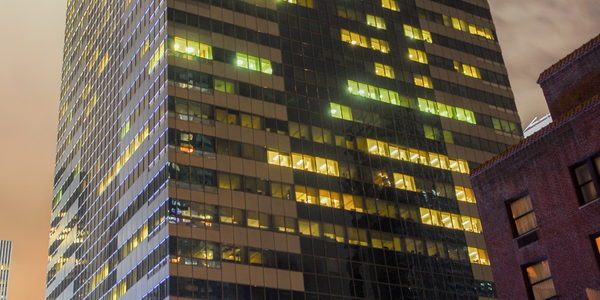
Case Study
Smooth Transition to Energy Savings
The building was equipped with four end-of-life Trane water cooled chillers, located in the basement. Johnson Controls installed four York water cooled centrifugal chillers with unit mounted variable speed drives and a total installed cooling capacity of 6,8 MW. Each chiller has a capacity of 1,6 MW (variable to 1.9MW depending upon condenser water temperatures). Johnson Controls needed to design the equipment in such way that it would fit the dimensional constraints of the existing plant area and plant access route but also the specific performance requirements of the client. Morgan Stanley required the chiller plant to match the building load profile, turn down to match the low load requirement when needed and provide an improvement in the Energy Efficiency Ratio across the entire operating range. Other requirements were a reduction in the chiller noise level to improve the working environment in the plant room and a wide operating envelope coupled with intelligent controls to allow possible variation in both flow rate and temperature. The latter was needed to leverage increased capacity from a reduced number of machines during the different installation phases and allow future enhancement to a variable primary flow system.
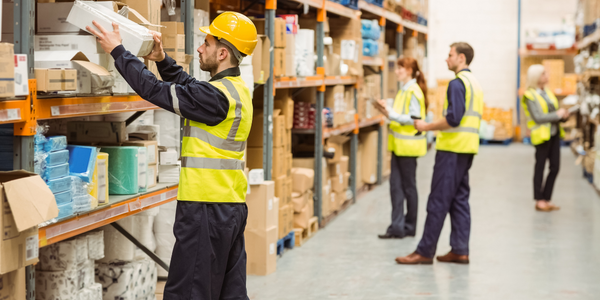
Case Study
Automated Pallet Labeling Solution for SPR Packaging
SPR Packaging, an American supplier of packaging solutions, was in search of an automated pallet labeling solution that could meet their immediate and future needs. They aimed to equip their lines with automatic printer applicators, but also required a solution that could interface with their accounting software. The challenge was to find a system that could read a 2D code on pallets at the stretch wrapper, track the pallet, and flag any pallets with unread barcodes for inspection. The pallets could be single or double stacked, and the system needed to be able to differentiate between the two. SPR Packaging sought a system integrator with extensive experience in advanced printing and tracking solutions to provide a complete traceability system.
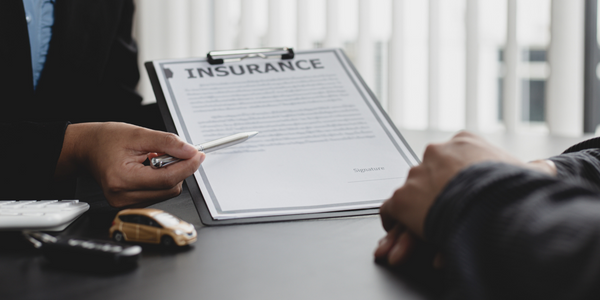
Case Study
Transforming insurance pricing while improving driver safety
The Internet of Things (IoT) is revolutionizing the car insurance industry on a scale not seen since the introduction of the car itself. For decades, premiums have been calculated using proxy-based risk assessment models and historical data. Today, a growing number of innovative companies such as Quebec-based Industrielle Alliance are moving to usage-based insurance (UBI) models, driven by the advancement of telematics technologies and smart tracking devices.
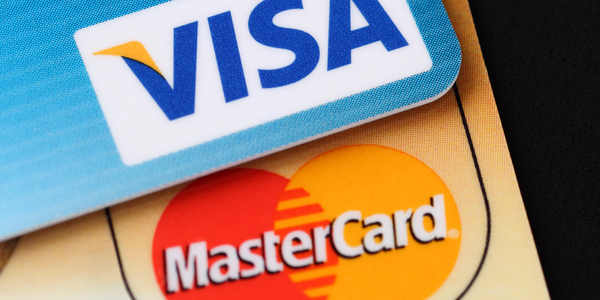
Case Study
MasterCard Improves Customer Experience Through Self-Service Data Prep
Derek Madison, Leader of Business Financial Support at MasterCard, oversees the validation of transactions and cash between two systems, whether they’re MasterCard owned or not. He was charged with identifying new ways to increase efficiency and improve MasterCard processes. At the outset, the 13-person team had to manually reconcile system interfaces using reports that resided on the company’s mainframe. Their first order of business each day was to print 20-30 individual, multi-page reports. Using a ruler to keep their place within each report, they would then hand-key the relevant data, line by line, into Excel for validation. “We’re talking about a task that took 40-80 hours each week,” recalls Madison, “As a growing company with rapidly expanding product offerings, we had to find a better way to prepare this data for analysis.”