Experimentation Automation
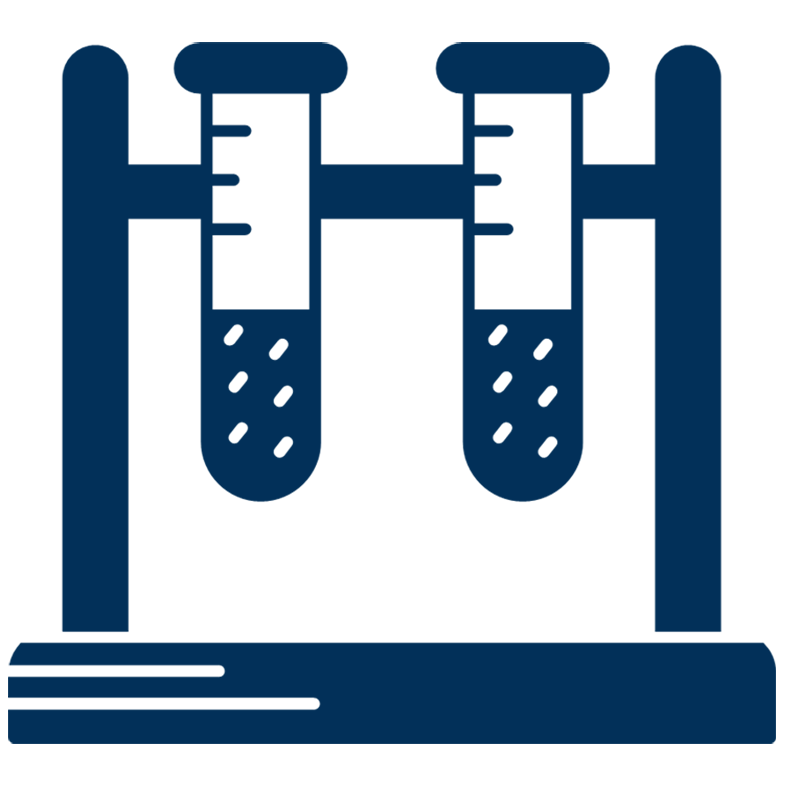
Automated experimentation encompasses all aspects of the automation of clusters of high throughput experiments in the fields of biomedicine and chemistry. Automation provides opportunities to conduct traditional experiments more efficiently and provides an alternative to the traditional experimental method in situations in which experimental variables are too numerous or complex to control. The most widely used application of laboratory automation technology is robotics. However, automated experimentation includes a wide range of laboratory instruments, devices such as autosamplers, software algorithms, and methodologies used to expedite and increase the efficiency of scientific research in laboratories.
Research and Development (R&D) Departments: Businesses invest in experimentation automation to accelerate innovation, reduce time-to-market, and improve product quality. R&D departments use automated experimentation platforms and tools to design experiments, collect data, and analyze results more efficiently, allowing them to explore a wider range of hypotheses and iterate on designs faster.
Product Development Teams: Product development teams leverage experimentation automation to optimize product features, user experience, and performance. By automating A/B testing, multivariate testing, and user feedback analysis, product development teams can make data-driven decisions, prioritize product enhancements, and drive continuous improvement throughout the product lifecycle.
Researchers and Scientists: Researchers and scientists value experimentation automation for its ability to streamline experimental workflows, reduce manual labor, and increase experimental throughput. They rely on automated experimentation platforms to design complex experiments, generate reproducible results, and uncover new insights more efficiently, enabling them to focus on higher-level analysis and interpretation.
Product Managers and Engineers: Product managers and engineers see experimentation automation as a strategic tool for driving product innovation, improving user satisfaction, and gaining competitive advantage. They use automated experimentation platforms to test product hypotheses, validate design decisions, and optimize product features based on user feedback and performance metrics, leading to faster iterations and better outcomes.
Automated Experimentation Platforms: Experimentation automation platforms provide a suite of tools and capabilities to design, execute, and analyze experiments efficiently. These platforms include experiment design tools, data collection modules, analysis algorithms, and visualization dashboards to streamline experimental workflows and accelerate discovery.
Sensor and Instrumentation Technology: Experimentation automation systems leverage sensor technology, data loggers, and digital instruments to collect real-time data on experimental variables, environmental conditions, and performance metrics. Sensors and instruments are integrated into experimental setups to monitor and control experimental parameters, ensuring reproducibility and accuracy.
Data Management and Analytics Software: Experimentation automation systems rely on data management and analytics software to store, process, and analyze experimental data. These software platforms include database systems, data processing pipelines, and machine learning algorithms to manage large volumes of experimental data, perform statistical analysis, and extract meaningful insights.
Experimental Data Collection: Experimentation automation systems collect real-time data on experimental variables, inputs, and outcomes using sensors, data loggers, and digital instruments. This data is stored in databases, data lakes, or cloud platforms for analysis and interpretation, allowing researchers and engineers to track experiment progress, monitor performance metrics, and identify trends over time.
Data Analysis and Visualization: Experimentation automation platforms leverage data analysis and visualization tools to process experimental data, perform statistical analysis, and generate insights. Researchers and engineers use data visualization techniques such as charts, graphs, and dashboards to visualize experimental results, communicate findings, and make data-driven decisions.
Hardware Installation: Deployment includes the installation of sensors, data acquisition systems, and automation equipment in laboratory settings, manufacturing facilities, or testing environments. Hardware installation may require calibration, testing, and validation to ensure accurate data collection and reliable operation during experiments.
Software Integration: Deployment involves integrating experimentation automation software with existing laboratory information management systems (LIMS), data analysis tools, and experimental control systems. Software integration enables seamless data exchange, workflow automation, and collaboration among researchers and engineers across different departments or locations.