Neptune.ai
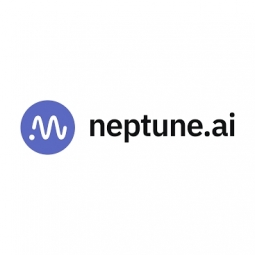
Overview
HQ Location
Poland
|
Year Founded
2017
|
Company Type
Private
|
Revenue
< $10m
|
Employees
51 - 200
|
Website
|
Company Description
Neptune is an experiment tracker for ML teams that struggle with debugging and reproducing experiments, sharing results, and messy model handover.
It offers a single place to track, compare, store, and collaborate on experiments so that Data Scientists can develop production-ready models faster and ML Engineers can access model artifacts instantly in order to deploy them to production.
IoT Snapshot
Neptune.ai is a provider of Industrial IoT analytics and modeling, infrastructure as a service (iaas), application infrastructure and middleware, robots, platform as a service (paas), and sensors technologies, and also active in the buildings, cement, construction and infrastructure, consumer goods, education, equipment and machinery, oil and gas, and retail industries.
Technologies
Use Cases
Functional Areas
Industries
Services
Technology Stack
Neptune.ai’s Technology Stack maps Neptune.ai’s participation in the analytics and modeling, infrastructure as a service (iaas), application infrastructure and middleware, robots, platform as a service (paas), and sensors IoT Technology stack.
-
Devices Layer
-
Edge Layer
-
Cloud Layer
-
Application Layer
-
Supporting Technologies
Technological Capability:
None
Minor
Moderate
Strong
Supplier missing?
Start adding your own!
Register with your work email and create a new supplier profile for your business.
Case Studies.
Case Study
Brainly's Integration with Amazon SageMaker and Neptune for Enhanced Machine Learning Capabilities
Brainly, a leading global learning platform, faced a challenge with their machine learning-powered feature, Snap to Solve. The feature allows users to upload a photo of a problem, which the system then detects and provides solutions for. The Visual Search team, responsible for the Visual Content Extraction (VICE) system of Snap to Solve, used Amazon SageMaker to run their computing workloads and serve their models. However, as the number of training runs on their large compute architectures increased, they found that their logs from Amazon SageMaker needed to be trackable and manageable to avoid workflow bottlenecks. They needed a tool that could scale regardless of the experiment volume. While they tried using SageMaker Experiments for tracking, they found the tracking UX and Python client unsatisfactory.
Case Study
Theta Tech AI: Enhancing Healthcare AI Systems with Neptune
Theta Tech AI, a company that builds customized artificial intelligence algorithms and front-end user interfaces for large-scale healthcare AI systems, faced several challenges in developing generalizable medical AI systems. The team had to manage thousands of experiments for large-scale parallel training workflows, which were run on GPU servers in AWS. However, they found that AWS CloudWatch Logs, their initial choice for monitoring the jobs, was inadequate for managing experiment logs. The team was unable to get experiment-relevant metrics from AWS CloudWatch Logs, debug problems with training jobs and experiments, integrate Optuna for hyperparameter optimization, and communicate the results of ML models to clients effectively.
Case Study
Optimizing Infrastructure Design with Continuum Industries' Optioneer Engine and Neptune
Continuum Industries, a company in the infrastructure industry, developed a product called Optioneer to automate and optimize the design of linear infrastructure assets. However, operating the Optioneer engine presented several challenges. The engine needed to be robust enough to handle different problems fed by different customers. Moreover, the company needed to ensure that the solutions provided by the engine were accurate and agreed upon by civil engineers. The team also had to constantly improve the optimization engine without breaking the algorithm. The nature of the problem they were trying to solve presented additional challenges. They could not automatically tell whether an algorithm output was correct or not. They needed a set of example problems that was representative of the kind of problem that the algorithm would be asked to solve in production. The team initially developed a custom solution to these problems, but it proved to be extremely clunky and complex to maintain.
Case Study
Leveraging Machine Learning to Analyze Impact of Promotional Campaigns on Sales
deepsense.ai, an AI-focused software services company, was tasked with a project for a leading Central and Eastern European food company. The project involved using machine learning to analyze the impact of promotional campaigns on sales. The food company runs various promotional campaigns for different products and wanted to create a model that predicts the number of sales per day for a given product on a promotional campaign. The challenge was the complexity of the data involving a large corpus of data sources, hundreds of different products, contractors, thousands of contractors’ clients, different promotion types, various promotion periods, overlapping promotions, and actions of the competition. It was also difficult to determine whether the sales increase was caused by any of the promotions applied, by the synergy between them, or it took place regardless of any campaigns.
Case Study
Waabi's Implementation of Neptune for Enhanced Experimentation Workflow and Resource Monitoring
Waabi, a company focused on developing the next generation of self-driving truck technology, faced a significant challenge in managing their large-scale experimentation workflow. Their Machine Learning teams, organized around different technical pillars, constantly launched experiments for different tasks, seeking model improvements by iteratively fine-tuning them and regularly comparing results against established benchmarks. The data involved in these experiments was diverse, including maps, LiDAR, camera, radar, inertial, and other sensor data. Keeping track of the data collected from these experiments and exporting it in an organized and shareable way became a challenge. The company also identified a lack of tooling for planning and building consistent benchmark datasets. They needed a solution that would allow them to share benchmark results in a constant place and format and retain data for later comparison after the end of a project.
Case Study
InstaDeep's BioAI Team Streamlines Experiment Management with Neptune
InstaDeep's BioAI team was faced with the challenge of managing numerous experiments for their DeepChain™ platform, a complex software for protein design. The team was dealing with scattered experiment logs, difficulty in sharing experiment results, and the burden of handling infrastructure and operations. The team needed a solution that was easy to use, could connect to TensorFlow and PyTorch logs, and was cost-effective. The challenge was to find a tool that could streamline their experiment management process, improve visibility of experiment logs, and facilitate collaboration among researchers and engineers.
Case Study
Optimizing Sports Data Analysis with IoT: A Case Study of ReSpo.Vision
ReSpo.Vision, a company specializing in sports data analysis, faced significant challenges in managing their machine learning (ML) pipelines at scale. The company uses computer vision and machine learning to extract 3D data from single-view camera sports broadcast videos, providing valuable insights to players, scouts, managers, clubs, and federations. Their ML team collects raw data, labels it, and adds new datasets to training and evaluation pipelines. However, as they scaled up the number of matches processed, the number of pipelines ran to build different models also increased, making it extremely hard to manage the workflow and debug pipeline failures. They had difficulty in debugging issues with the experiment results, figuring out if their pipelines all finished successfully, and comparing the results of each run to the previous runs. Additionally, it was problematic to know what dataset or parameters were used for each experiment run. They needed a better way to manage their pipeline runs and make the best use of their resources.
Case Study
Implementing Neptune for Efficient Machine Learning in Bioinformatics: A Case Study of ailslab
ailslab, a small bioinformatics research group, is dedicated to building machine learning models to predict cardiovascular disease development using clinical, imaging, and genetics data. The research process is intense, requiring a custom infrastructure to extract features from various data types, including Electronic Health Records (EHR), time-to-event data, images, structured data, and ECG. The goal is to create precise machine learning models for risk stratification for primary cardiovascular prevention. However, as the team grew, collaboration became more challenging, and new problems began to emerge. These included issues with data privacy, workflow standardization, feature and model selection, experiment management, and information logging.
Case Study
Hypefactors: Enhancing Media Intelligence with IoT and Machine Learning
Hypefactors, a technology company specializing in media intelligence and reputation tracking, faced a significant challenge in managing their data pipelines. These pipelines monitor a wide range of media, including social media, print, television, and radio, to analyze changes in their customers' brand reputation. The process involves gathering data from various sources and enriching it with machine learning (ML) features. However, as the company expanded its operations and started working on more complex ML problems, they encountered difficulties in tracking their experiments. Initially, the team used Slack for collaboration and personal notes/files for storing training metadata and model artifacts. However, as the number of models, features, and team members increased, this method became inefficient and created structural bottlenecks.
Case Study
Streamlining Research and Project Management in AI and ML with Neptune: A Case Study at TH Köln
TH Köln, Germany’s largest University of Applied Sciences in the Electrical Engineering Department, was facing significant challenges in managing large-scale research projects. The department, which focuses on meta-learning research with standard ML frameworks such as TensorFlow and PyTorch, was struggling with experiment-tracking across multiple servers. The team was manually creating CSV files to record details generated during the experiment run such as loss or f2 score. Additionally, there were separate files with hyperparameters and other configurations. This manual management of multiple files made the analysis of past experiments extremely challenging and prone to errors. The team was also facing issues with multi-server project management, access control management, result comparison and presentation, and loss of experiment history when students left.
Case Study
Zoined: Enhancing Retail and Hospitality Analytics with Neptune
Zoined, a company offering cloud-based Retail and Hospitality Analytics, faced a significant challenge in tracking and managing experiments, especially with a small team of scientists and engineers. The company's data scientist, Kha, was solely responsible for the forecasting pipeline, making experiment tracking a tedious manual task. Kha was dealing with large data frames with forecasts that needed to be logged alongside their experiments. He also needed a way to visualize results for complete and intermediate experiments to enhance efficiency. The team initially tried using Splunk for experiment tracking, but it proved to be intimidating, difficult for visualizing logged values, and expensive. The next solution, MLflow, presented issues with hosting options, was compute-intensive, and had problems with auto scaling. It also made collaboration difficult as sharing experiments was not straightforward.