Customer Company Size
Large Corporate
Region
- America
Country
- United States
Product
- Intermedix technology-enabled services
- Dataiku DSS
Tech Stack
- Dataiku DSS
- Python
- R
Implementation Scale
- Enterprise-wide Deployment
Impact Metrics
- Cost Savings
- Productivity Improvements
Technology Category
- Analytics & Modeling - Predictive Analytics
Applicable Industries
- Healthcare & Hospitals
Applicable Functions
- Business Operation
Use Cases
- Predictive Replenishment
Services
- Data Science Services
About The Customer
Intermedix, founded in 2002, delivers technology-enabled services and SaaS solutions to healthcare providers, government agencies, and corporations. As a leading provider of technology-enabled solutions for the global health and safety net, Intermedix supports more than 15,000 healthcare providers with practice management, revenue cycle management, and data analytic tools. The company connects more than 95 percent of the U.S. population with crisis management and emergency preparedness technologies. Intermedix is now helping more than 50 clinics reduce costs related to patient no-shows.
The Challenge
The healthcare industry is grappling with a high rate of patient no-shows, with studies indicating that 5-10% of scheduled patients miss their appointments. This has a significant impact on the financial health of healthcare organizations and their ability to care for other patients. Primary care physicians lose an average revenue of $228 for every no-show, and the lost revenue for specialists is even higher. When a patient misses an appointment, overhead costs including staffing, insurance, and utilities are not reimbursed. Cancellations with primary care physicians also impact the number of necessary specialist referrals those physicians can make. Combined, these factors contribute to significant revenue loss for physicians. To help minimize the occurrence of no-shows and thus reduce associated costs, Intermedix decided to develop and operationalize a no-show predictor that would assist office managers in scheduling appointments.
The Solution
Intermedix introduced Dataiku DSS to their data science team to develop and operationalize a no-show predictor. The team set up the automatic ingestion and crunching of historical, appointment, and demographic patient data. The data scientists built a predictive model that scores individual patients based on the probability of them missing an appointment for an allocated time slot. This output is automatically fed directly to each individual clinic several times per week depending on demand. Office managers and schedulers can use this predictive report to optimize physician schedules and patient appointments. The development and deployment of such an application to cover site-specific patterns would typically take 3+ months. However, with Dataiku DSS, Intermedix’s data science team was able to prototype and deliver the solution in just one month.
Operational Impact
Quantitative Benefit
Case Study missing?
Start adding your own!
Register with your work email and create a new case study profile for your business.
Related Case Studies.
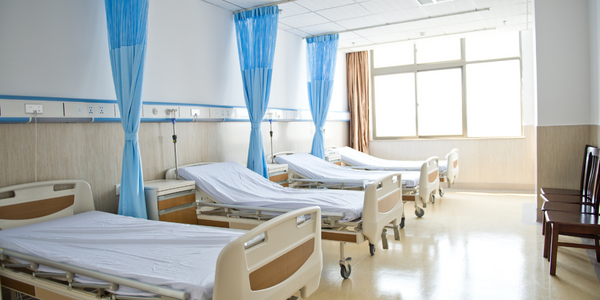
Case Study
Hospital Inventory Management
The hospital supply chain team is responsible for ensuring that the right medical supplies are readily available to clinicians when and where needed, and to do so in the most efficient manner possible. However, many of the systems and processes in use at the cancer center for supply chain management were not best suited to support these goals. Barcoding technology, a commonly used method for inventory management of medical supplies, is labor intensive, time consuming, does not provide real-time visibility into inventory levels and can be prone to error. Consequently, the lack of accurate and real-time visibility into inventory levels across multiple supply rooms in multiple hospital facilities creates additional inefficiency in the system causing over-ordering, hoarding, and wasted supplies. Other sources of waste and cost were also identified as candidates for improvement. Existing systems and processes did not provide adequate security for high-cost inventory within the hospital, which was another driver of cost. A lack of visibility into expiration dates for supplies resulted in supplies being wasted due to past expiry dates. Storage of supplies was also a key consideration given the location of the cancer center’s facilities in a dense urban setting, where space is always at a premium. In order to address the challenges outlined above, the hospital sought a solution that would provide real-time inventory information with high levels of accuracy, reduce the level of manual effort required and enable data driven decision making to ensure that the right supplies were readily available to clinicians in the right location at the right time.
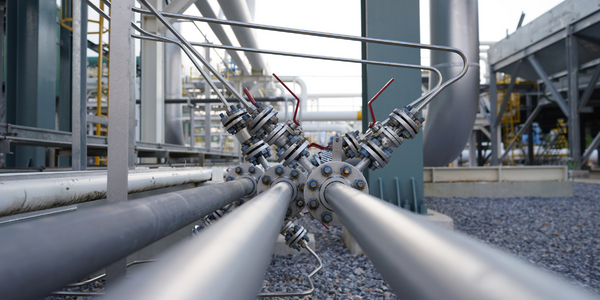
Case Study
Gas Pipeline Monitoring System for Hospitals
This system integrator focuses on providing centralized gas pipeline monitoring systems for hospitals. The service they provide makes it possible for hospitals to reduce both maintenance and labor costs. Since hospitals may not have an existing network suitable for this type of system, GPRS communication provides an easy and ready-to-use solution for remote, distributed monitoring systems System Requirements - GPRS communication - Seamless connection with SCADA software - Simple, front-end control capability - Expandable I/O channels - Combine AI, DI, and DO channels
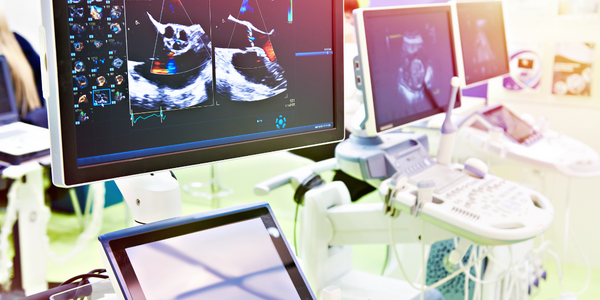
Case Study
Driving Digital Transformations for Vitro Diagnostic Medical Devices
Diagnostic devices play a vital role in helping to improve healthcare delivery. In fact, an estimated 60 percent of the world’s medical decisions are made with support from in vitrodiagnostics (IVD) solutions, such as those provided by Roche Diagnostics, an industry leader. As the demand for medical diagnostic services grows rapidly in hospitals and clinics across China, so does the market for IVD solutions. In addition, the typically high cost of these diagnostic devices means that comprehensive post-sales services are needed. Wanteed to improve three portions of thr IVD:1. Remotely monitor and manage IVD devices as fixed assets.2. Optimizing device availability with predictive maintenance.3. Recommending the best IVD solution for a customer’s needs.
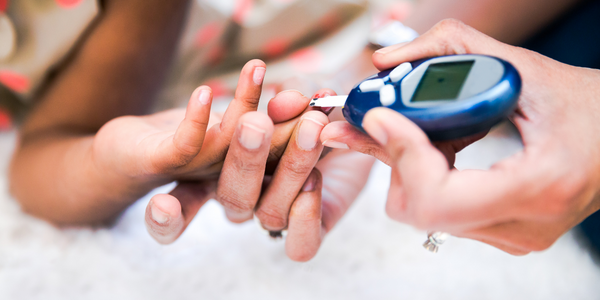
Case Study
HaemoCloud Global Blood Management System
1) Deliver a connected digital product system to protect and increase the differentiated value of Haemonetics blood and plasma solutions. 2) Improve patient outcomes by increasing the efficiency of blood supply flows. 3) Navigate and satisfy a complex web of global regulatory compliance requirements. 4) Reduce costly and labor-intensive maintenance procedures.
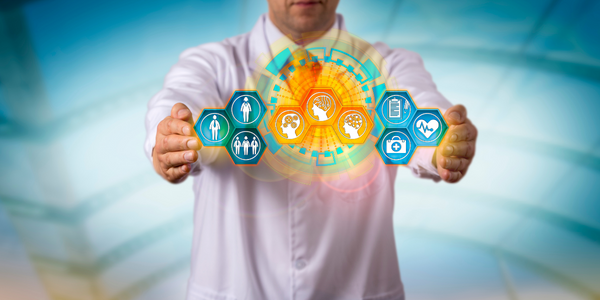
Case Study
Harnessing real-time data to give a holistic picture of patient health
Every day, vast quantities of data are collected about patients as they pass through health service organizations—from operational data such as treatment history and medications to physiological data captured by medical devices. The insights hidden within this treasure trove of data can be used to support more personalized treatments, more accurate diagnosis and more advanced preparative care. But since the information is generated faster than most organizations can consume it, unlocking the power of this big data can be a struggle. This type of predictive approach not only improves patient care—it also helps to reduce costs, because in the healthcare industry, prevention is almost always more cost-effective than treatment. However, collecting, analyzing and presenting these data-streams in a way that clinicians can easily understand can pose a significant technical challenge.