- Functional Applications - Manufacturing Execution Systems (MES)
- Automotive
- Additive Manufacturing
- Manufacturing Process Simulation
The customer in this case study is BMW Group, a global manufacturer of automobiles and motorcycles. BMW faces the challenge of optimizing their manufacturing plant scheduling to meet production targets while minimizing idle time. This involves a complex set of variables and constraints, including different production rates across shops, discrete shift schedules, and the need to prevent overflows and shortages in the buffers between manufacturing steps. The company is a member of MIT’s The Center for Quantum Engineering (CQE), where it collaborates with other members to apply advanced technologies like generative AI to solve complex problems.
Global manufacturers like BMW face a complex optimization problem: scheduling their workers to achieve production targets while minimizing idle hours. The challenge lies in the wide range of possible configurations and numerous constraints. Different shops have varying production rates, and each has their own discrete set of shift schedules. Furthermore, manufacturers need to prevent overflows and shortages in the buffers between steps in the manufacturing process. The complexity of the problem is further compounded by the need to optimize across multiple variables and constraints, making it a difficult task to solve using traditional methods.
Zapata and BMW Group, as part of their membership in MIT’s The Center for Quantum Engineering (CQE), collaborated to apply generative AI techniques to BMW’s plant scheduling optimization problem. They trained a quantum-inspired generative model on the best solutions generated by existing state-of-the-art solvers. This generative model then generated new, previously unconsidered solutions. This approach is called Generator-Enhanced Optimization (GEO). Using Zapata's computational workflow platform, Orquestra®, they ran about one million optimization runs cycling through dozens of different categories of algorithms, problem configurations and optimizer solutions to benchmark their performance against each other. They identified the best algorithm to solve each problem configuration, whether it was GEO or a conventional solver. GEO performed particularly well in configurations with large spaces of possible solutions, outperforming all other solvers in the configuration with the largest solution space.
Case Study missing?
Start adding your own!
Register with your work email and create a new case study profile for your business.
Related Case Studies.
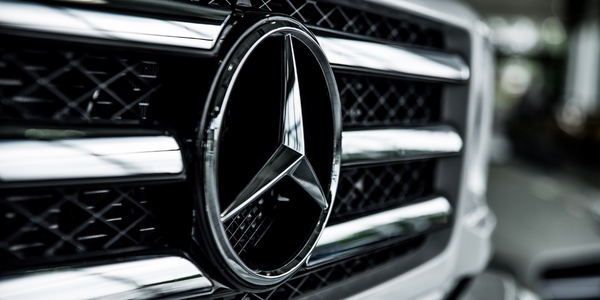
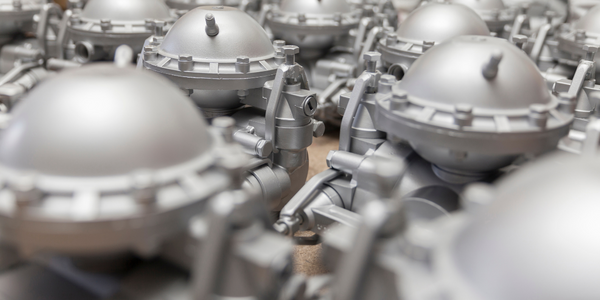