- Analytics & Modeling - Machine Learning
- Application Infrastructure & Middleware - Middleware, SDKs & Libraries
- Cement
- Education
- Logistics & Transportation
- Last Mile Delivery
- Leasing Finance Automation
- Data Science Services
- Training
VTS is a commercial real estate leasing and asset management software and data company. It was founded by real estate professionals who have experienced the challenges facing today’s landlords and brokers. VTS delivers an easy-to-use, intuitive workflow and insights platform that empowers commercial real estate professionals to work smarter, not harder. Over 12 billion square feet of commercial real estate is managed on the VTS platform. Their mission is to be the decision-making platform for the commercial real estate industry, where real-time insights come to life.
VTS, a commercial real estate leasing and asset management software and data company, aimed to become the decision-making platform for the commercial real estate industry. To achieve this, they wanted to efficiently productionize Machine Learning (ML) models and build new models iteratively using AWS services. Their goal was to accelerate the time to market for ML applications, reduce human errors, and lessen the effort from their Data Science (DS) team. One of the ML models they prototyped was designed to predict leasing deal outcomes. However, they faced challenges in integrating this predictive model into the core user experience. While their data scientists were capable of delivering the model in ad hoc environments, they found it difficult to deploy the model in production using the existing infrastructure of the VTS platform. In essence, VTS had excellent data scientists but lacked the necessary AWS and MLOps expertise to complete the task.
To overcome these challenges, VTS partnered with Provectus, an AWS Premier Consulting Partner. Provectus delivered a template-based solution that enabled VTS data scientists to more easily create Amazon SageMaker jobs, pipelines, endpoints, and other AWS resources. They designed, built, and delivered VTS-specific templates and SDK to create jobs, pipelines, and endpoints in Amazon SageMaker and other AWS services. The first set of templates provided the foundation for refactoring data ingestion, data preprocessing, feature engineering, and model training scripts into Amazon SageMaker jobs. The delivered SDK, called VTS ML Commons SDK, extended Amazon SageMaker SDK with components tailored for VTS environments. The second set of templates simplified connecting different workflow steps into Amazon SageMaker pipelines for training, batch-mode, and real-time model serving, and integration with other AWS services like Amazon EventBridge or AWS Lambda. Provectus also prepared the required documentation and held several knowledge transfer sessions with VTS ML engineers.
Case Study missing?
Start adding your own!
Register with your work email and create a new case study profile for your business.
Related Case Studies.
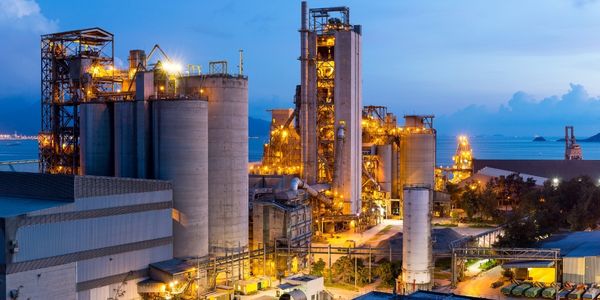
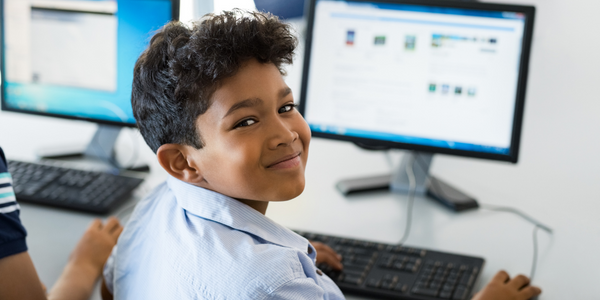
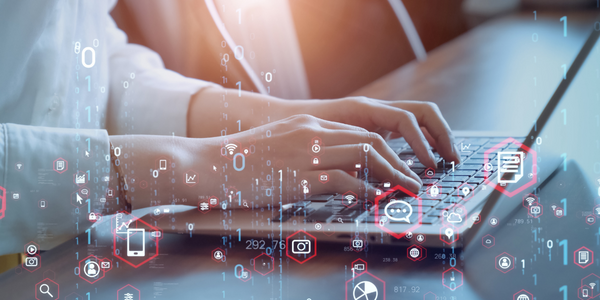
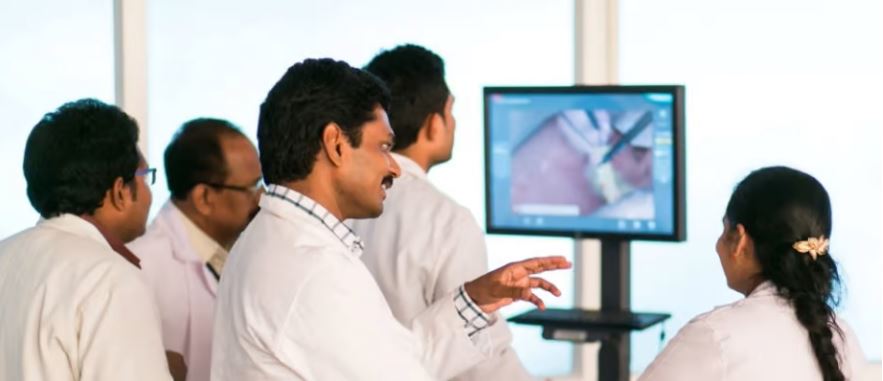
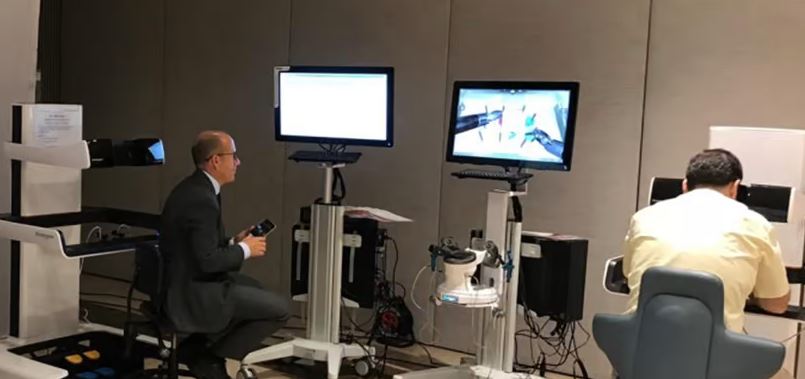