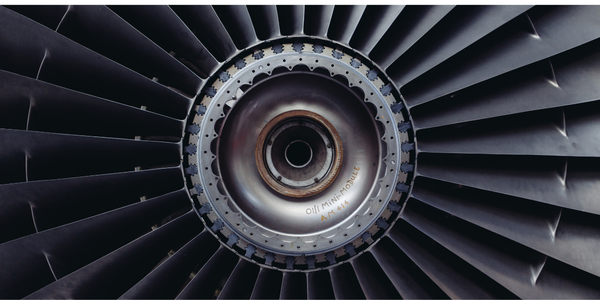
- Analytics & Modeling - Machine Learning
- Analytics & Modeling - Predictive Analytics
- Application Infrastructure & Middleware - Data Visualization
- Equipment & Machinery
- Maintenance
- Predictive Maintenance
In late 2015, a deployed combustion turbine experienced a row two vane failure, which caused massive secondary damage to the compressor, resulting in nearly two months of downtime and up to $30M in repairs costs and lost opportunity. This failure, though rare, is representative of typical catastrophic events that are very difficult to catch. Though the onsite plant operations team had been monitoring the asset, this specific failure mode was previously unknown and very nuanced, and existing alarms did not have enough information for SMEs to properly diagnose it in time.
The OEM decided to evaluate SparkCognition’s predictive analytics solution, SparkPredict®, with the following objectives:
1. Demonstrate the ability to detect and distinguish operational and anomalous online steady-state conditions based on blind data provided from the turbine.
2. Provide additional insights about the key contributing factors to the underlying anomalies.
3. Provide a UI that interfaces to live streaming data from the asset.
Over the course of one quarter, SparkCognition developed, trained, and validated high-performance machine learning models for each steady and transient operating mode, using two years’ worth of data from the combustion turbine in question. SparkPredict’s data science team built these unique models by leveraging proprietary internal tools and automated model building capabilities to execute quickly and effectively.
Using these methods, the team first cleansed the data to prepare for modelling. They reduced the original set of over 400 variables down to the roughly 250 which best represented the data set. Then, using an unsupervised clustering approach, they identified all the various operating states of the turbine, working with the customer SME to label each known failure event cluster. Lastly, the model was validated with actual failure event data from the OEM.
Case Study missing?
Start adding your own!
Register with your work email and create a new case study profile for your business.
Related Case Studies.
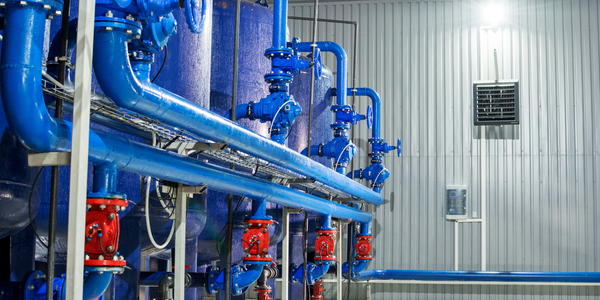
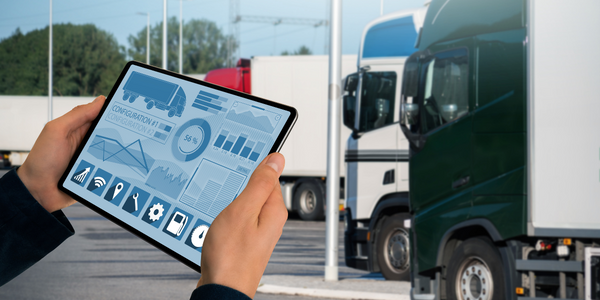
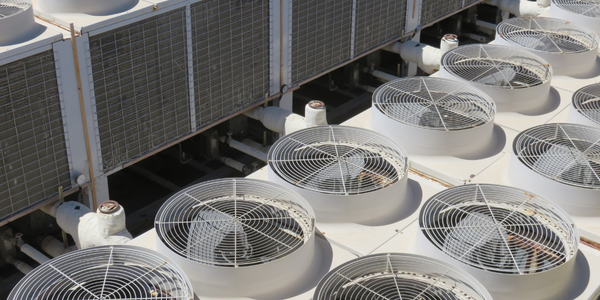
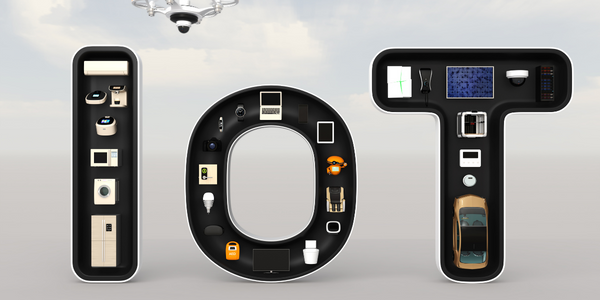
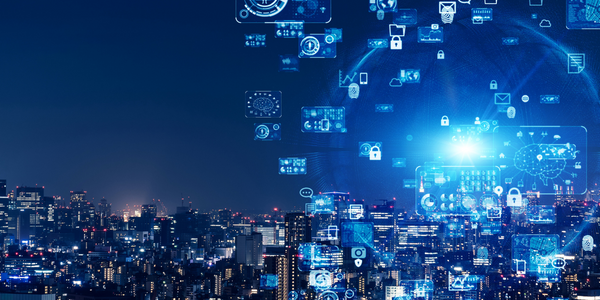
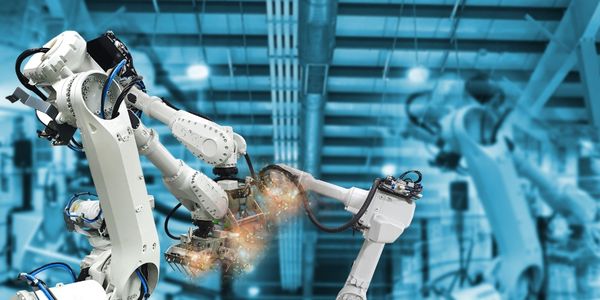