Customer Company Size
Mid-size Company
Region
- America
Country
- United States
Product
- Consumer Insights & Analytics
Tech Stack
- Data Analytics
- CRM
Implementation Scale
- Enterprise-wide Deployment
Impact Metrics
- Customer Satisfaction
- Brand Awareness
Technology Category
- Analytics & Modeling - Big Data Analytics
Applicable Industries
- Retail
Applicable Functions
- Sales & Marketing
- Business Operation
Use Cases
- Predictive Replenishment
Services
- Data Science Services
About The Customer
The customer is a national retailer of modern American-made home furnishings and accessories. Founded in 1980, the company has a passion for helping customers furnish homes they love. Their philosophy is that great design should be beautiful, affordable, and long-lasting. The company's core values include American made, sustainable design, natural materials, value, and exceptional customer service. They currently have 12 store locations and plan to open 2 additional stores this year in Boston, MA, and New York City.
The Challenge
The national retailer of modern American-made home furnishings and accessories was facing challenges in understanding when to grow, how to grow, who to grow, and where to grow. They were struggling with identifying the right time in the buying cycle to introduce consumers to their brand. Their customer acquisition strategy was not data-driven, leading to an undifferentiated approach where all customers were treated the same. They were also unsure about their future consumer and how to reach them. Lastly, their retail expansion goals were not aligned with their customer acquisition and development strategy.
The Solution
The company decided to leverage analytics to address their challenges. They used data to identify key times in the buying cycle when consumers are most likely to be in the market for a furniture purchase. This allowed them to synchronize their marketing messages for optimal timing. For customer acquisition, they segmented their preferred audiences and learned everything they could about each audience. This led to a multi-channel and multi-touch marketing perspective. To identify their future consumer, they looked at factors such as age, education, location, marital status, and generation. They then translated these characteristics into Mosaic® USA segments. For retail expansion, they incorporated their customer acquisition and retention strategy into the market planning process. They used CRM data to gain insights into the characteristics of customers within proximity to new site locations.
Operational Impact
Case Study missing?
Start adding your own!
Register with your work email and create a new case study profile for your business.
Related Case Studies.
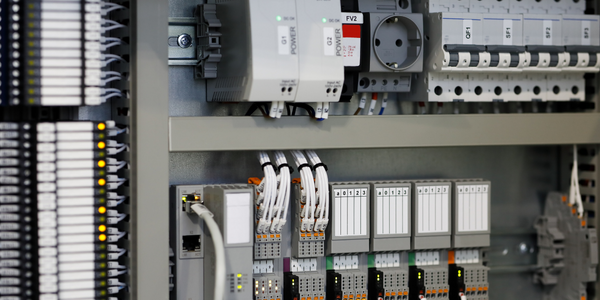
Case Study
Improving Production Line Efficiency with Ethernet Micro RTU Controller
Moxa was asked to provide a connectivity solution for one of the world's leading cosmetics companies. This multinational corporation, with retail presence in 130 countries, 23 global braches, and over 66,000 employees, sought to improve the efficiency of their production process by migrating from manual monitoring to an automatic productivity monitoring system. The production line was being monitored by ABB Real-TPI, a factory information system that offers data collection and analysis to improve plant efficiency. Due to software limitations, the customer needed an OPC server and a corresponding I/O solution to collect data from additional sensor devices for the Real-TPI system. The goal is to enable the factory information system to more thoroughly collect data from every corner of the production line. This will improve its ability to measure Overall Equipment Effectiveness (OEE) and translate into increased production efficiencies. System Requirements • Instant status updates while still consuming minimal bandwidth to relieve strain on limited factory networks • Interoperable with ABB Real-TPI • Small form factor appropriate for deployment where space is scarce • Remote software management and configuration to simplify operations
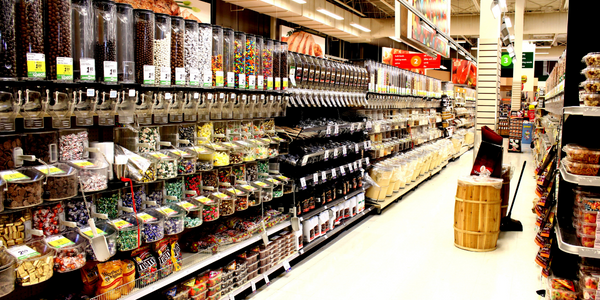
Case Study
How Sirqul’s IoT Platform is Crafting Carrefour’s New In-Store Experiences
Carrefour Taiwan’s goal is to be completely digital by end of 2018. Out-dated manual methods for analysis and assumptions limited Carrefour’s ability to change the customer experience and were void of real-time decision-making capabilities. Rather than relying solely on sales data, assumptions, and disparate systems, Carrefour Taiwan’s CEO led an initiative to find a connected IoT solution that could give the team the ability to make real-time changes and more informed decisions. Prior to implementing, Carrefour struggled to address their conversion rates and did not have the proper insights into the customer decision-making process nor how to make an immediate impact without losing customer confidence.
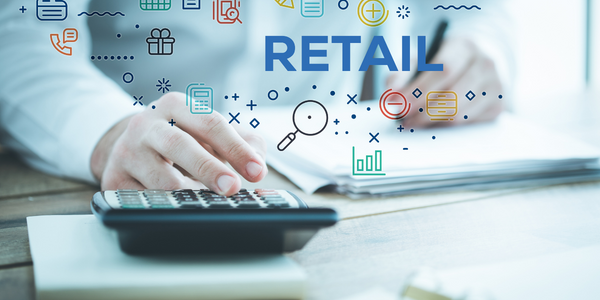
Case Study
Digital Retail Security Solutions
Sennco wanted to help its retail customers increase sales and profits by developing an innovative alarm system as opposed to conventional connected alarms that are permanently tethered to display products. These traditional security systems were cumbersome and intrusive to the customer shopping experience. Additionally, they provided no useful data or analytics.
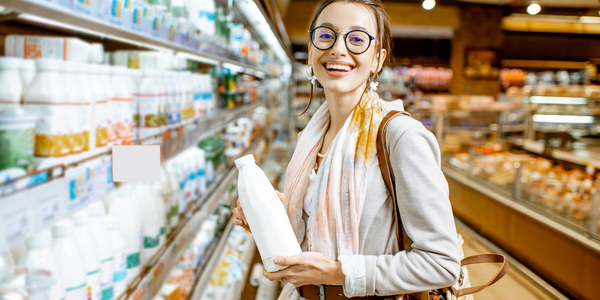
Case Study
Ensures Cold Milk in Your Supermarket
As of 2014, AK-Centralen has over 1,500 Danish supermarkets equipped, and utilizes 16 operators, and is open 24 hours a day, 365 days a year. AK-Centralen needed the ability to monitor the cooling alarms from around the country, 24 hours a day, 365 days a year. Each and every time the door to a milk cooler or a freezer does not close properly, an alarm goes off on a computer screen in a control building in southwestern Odense. This type of alarm will go off approximately 140,000 times per year, equating to roughly 400 alarms in a 24-hour period. Should an alarm go off, then there is only a limited amount of time to act before dairy products or frozen pizza must be disposed of, and this type of waste can quickly start to cost a supermarket a great deal of money.
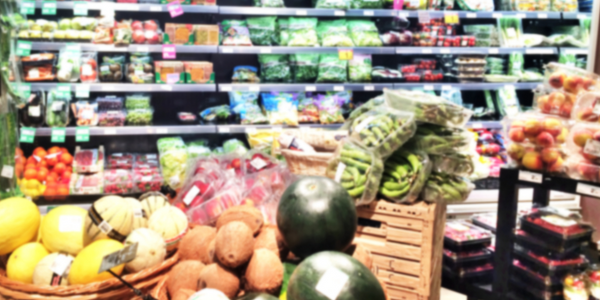
Case Study
Supermarket Energy Savings
The client had previously deployed a one-meter-per-store monitoring program. Given the manner in which energy consumption changes with external temperature, hour of the day, day of week and month of year, a single meter solution lacked the ability to detect the difference between a true problem and a changing store environment. Most importantly, a single meter solution could never identify root cause of energy consumption changes. This approach never reduced the number of truck-rolls or man-hours required to find and resolve issues.